Explore the key findings below or download the full report.
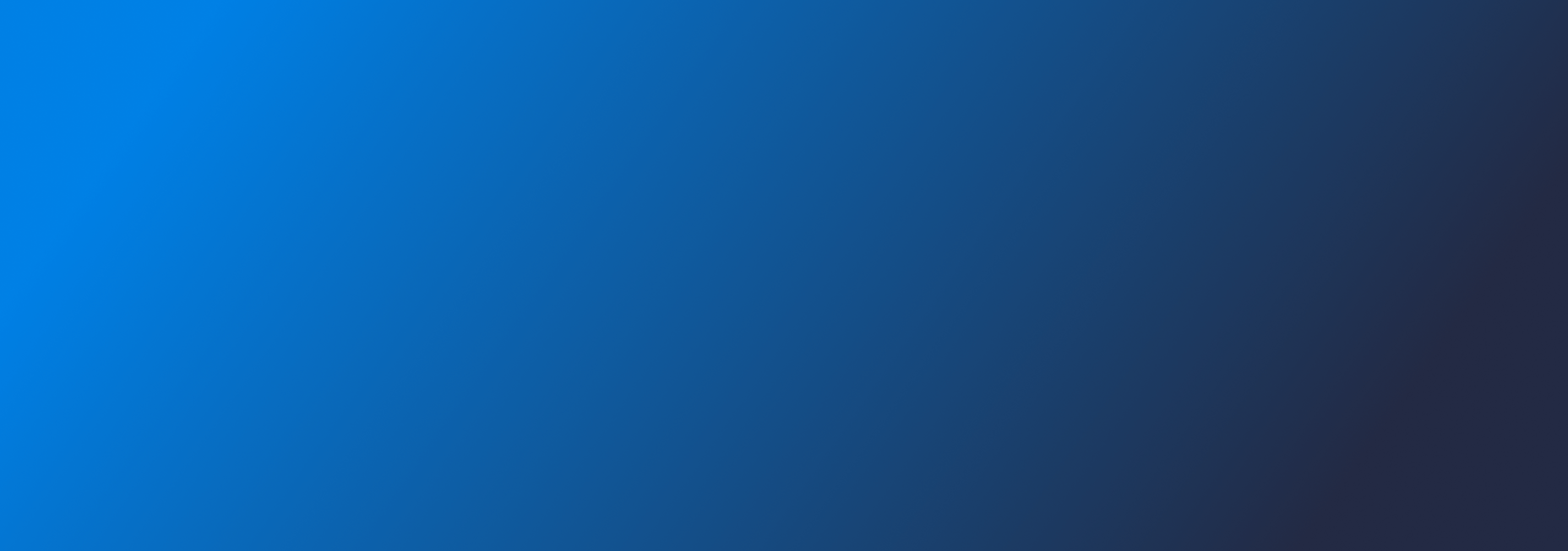
Evident AI Outcomes Report
Towards a benchmark for AI outcomes in banking
The Evident AI Outcomes Report examines how banks are setting themselves up to drive, and accelerate, value from AI. It marks the first step towards benchmarking AI outcomes across the sector. The report covers:
Use Case
ROI
Ideation
Delivery
Key Findings
1
The last year has seen a step-change increase in ambition and investment in AI across the banking sector.
Banks are generating more ideas for AI use cases than ever before. Working out how to scale up use cases, deliver value, and orchestrate the AI activities across the company has, in many banks, become the mandate for newly established group AI leadership teams. These teams are focused on building capabilities across five priority areas.
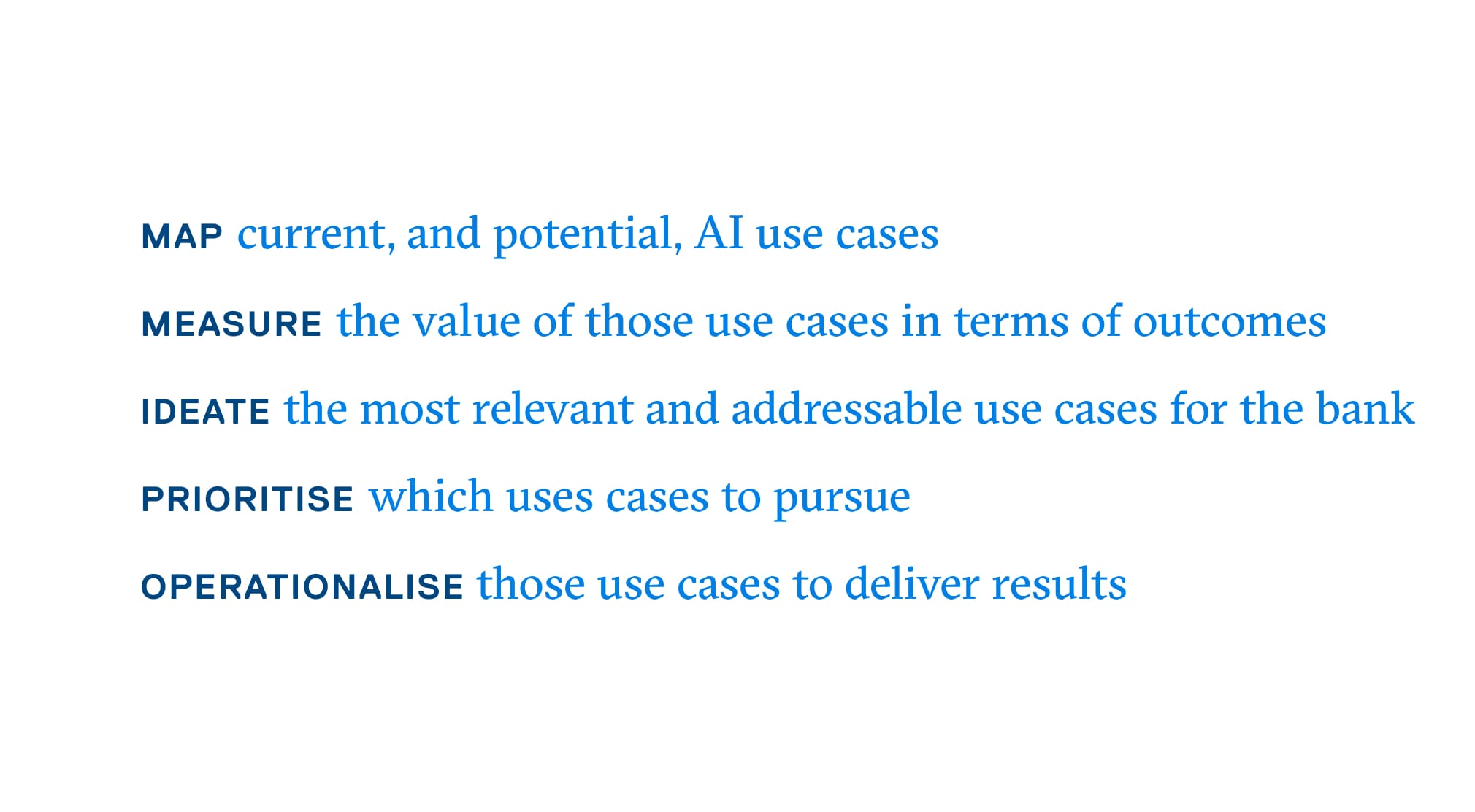
2
Map | Use cases are the building blocks of adoption within banks.
The exact definition of a use case can vary between banks. However, within a bank there should be consistent and standardised classification and terminology. Depending on how a bank itemises its use cases, it may report having tens, hundreds, or thousands across the organisation.
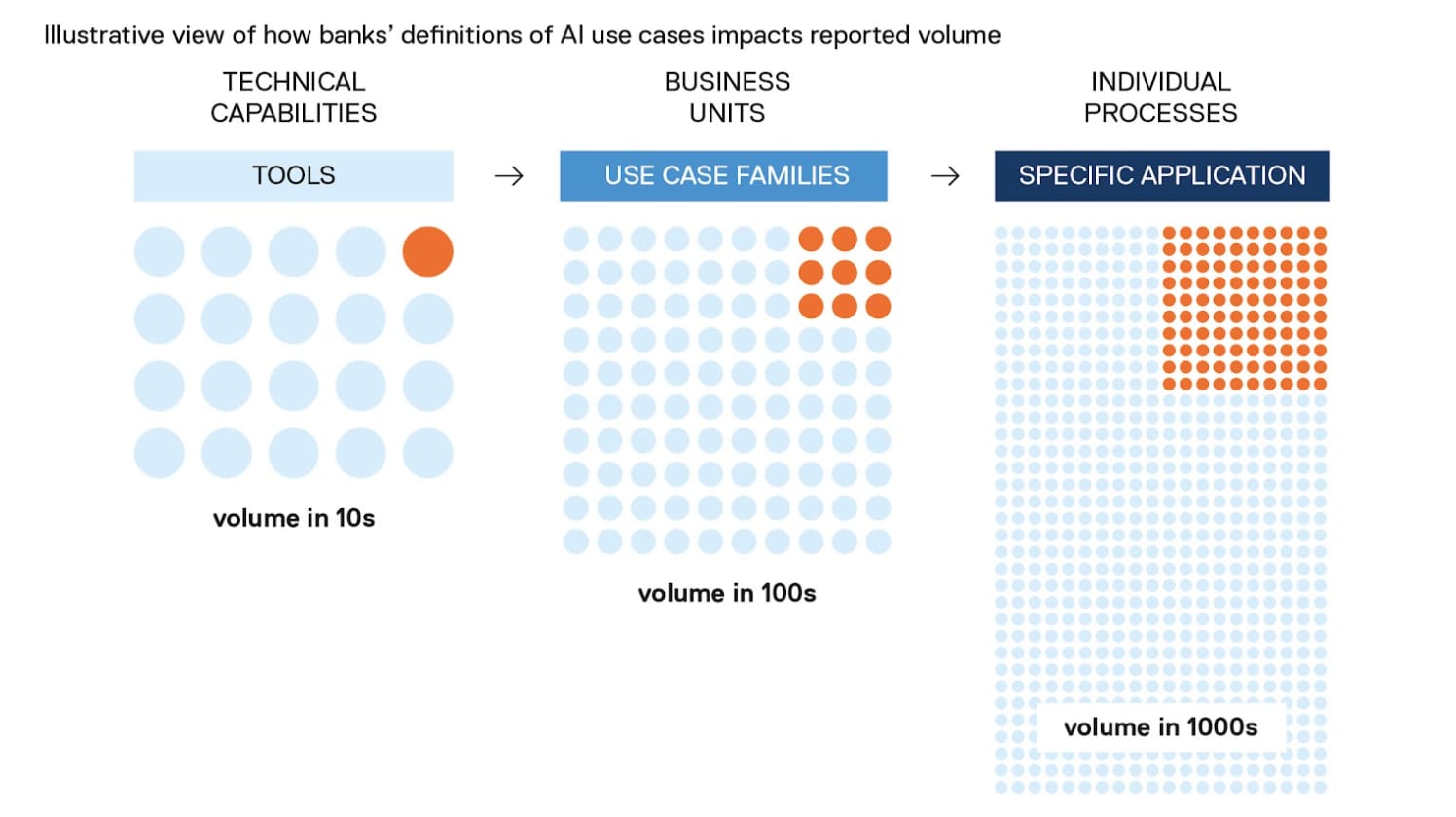
3
Measure | Banks need a methodology and process to measure, track and report on the value created by AI use cases.
Shareholders and senior leaders are increasingly demanding tangible outcomes from AI investments. Banks need a common methodology and process to measure, track and report on the value created by their existing (and future) AI use cases.
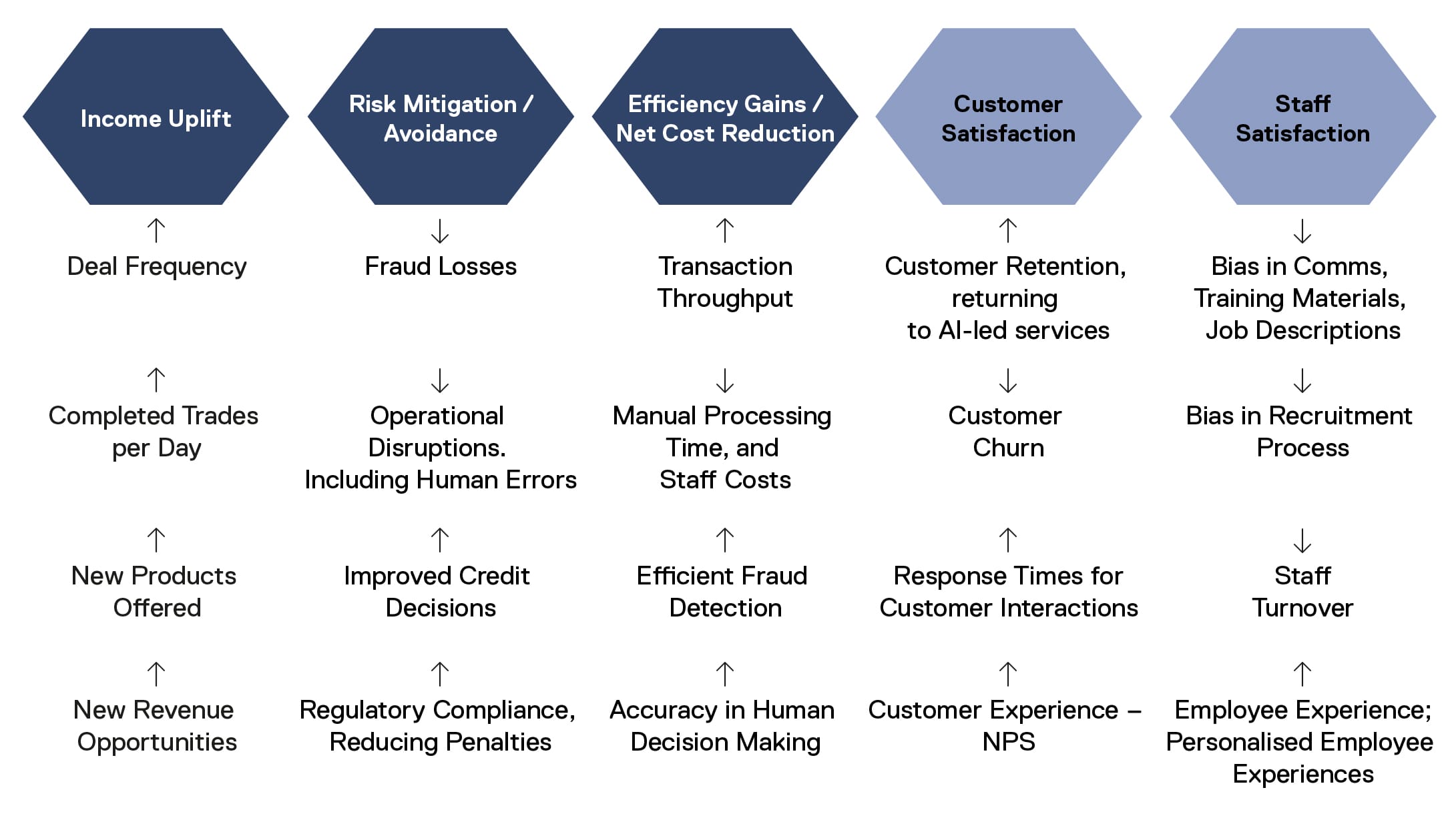
4
Ideate | Use cases start with a problem that needs to be solved.
The best use cases are intimately tied to business problems, and ChatGPT has led to the proliferation of ideas of AI use cases like never before. Leading AI teams are investing in initiatives to fuel and harness this bank-wide AI ideation.
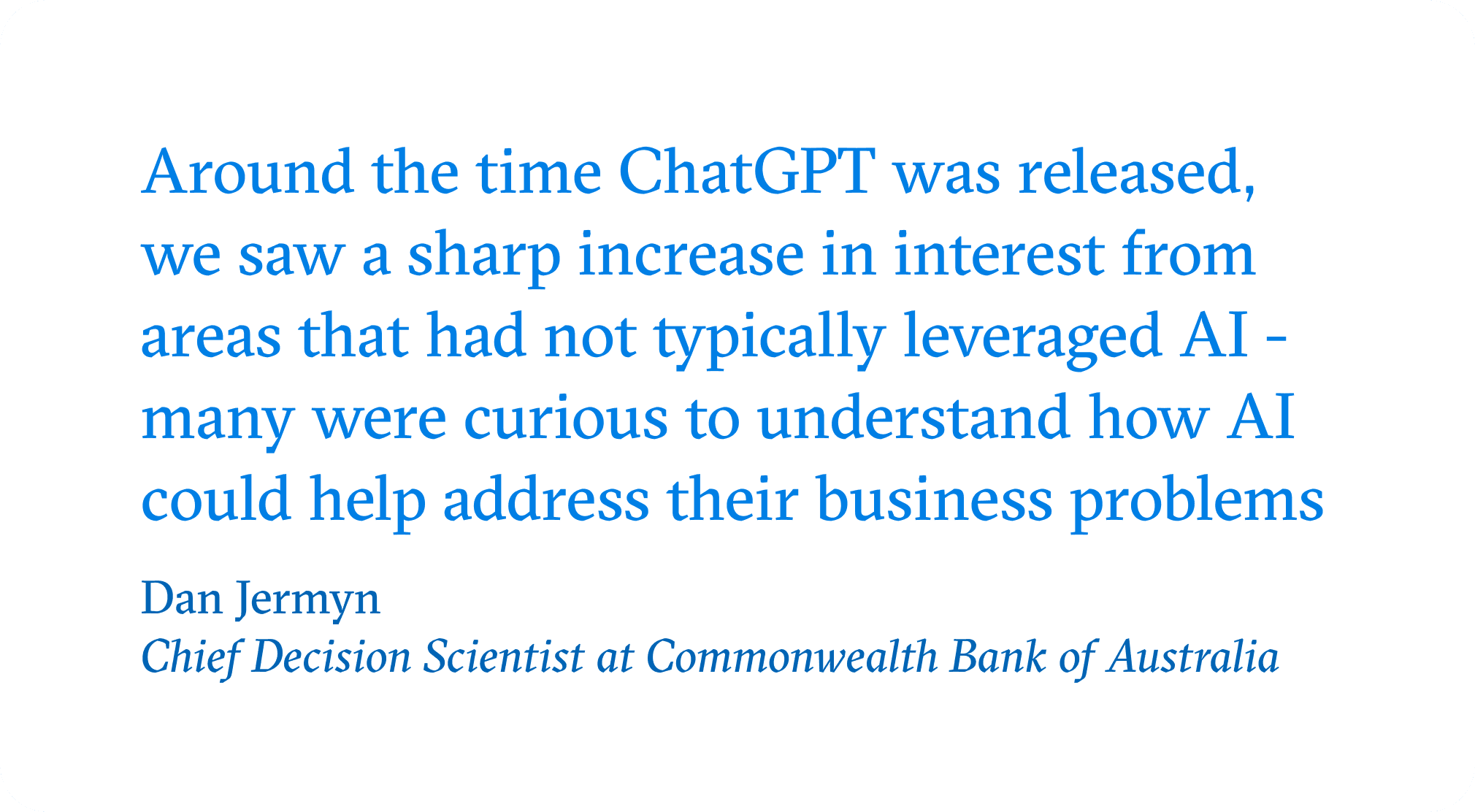
5
Prioritise | Banks need to be able to prioritise these use cases if they are not to drown in the sea of possibilities.
There may be few limits to the opportunities offered by AI - but delivery resources are always constrained. Banks need a robust, standardised and aligned process to prioritise AI use cases for delivery. This has to cover ROI, operational capacity, risk and governance issues.
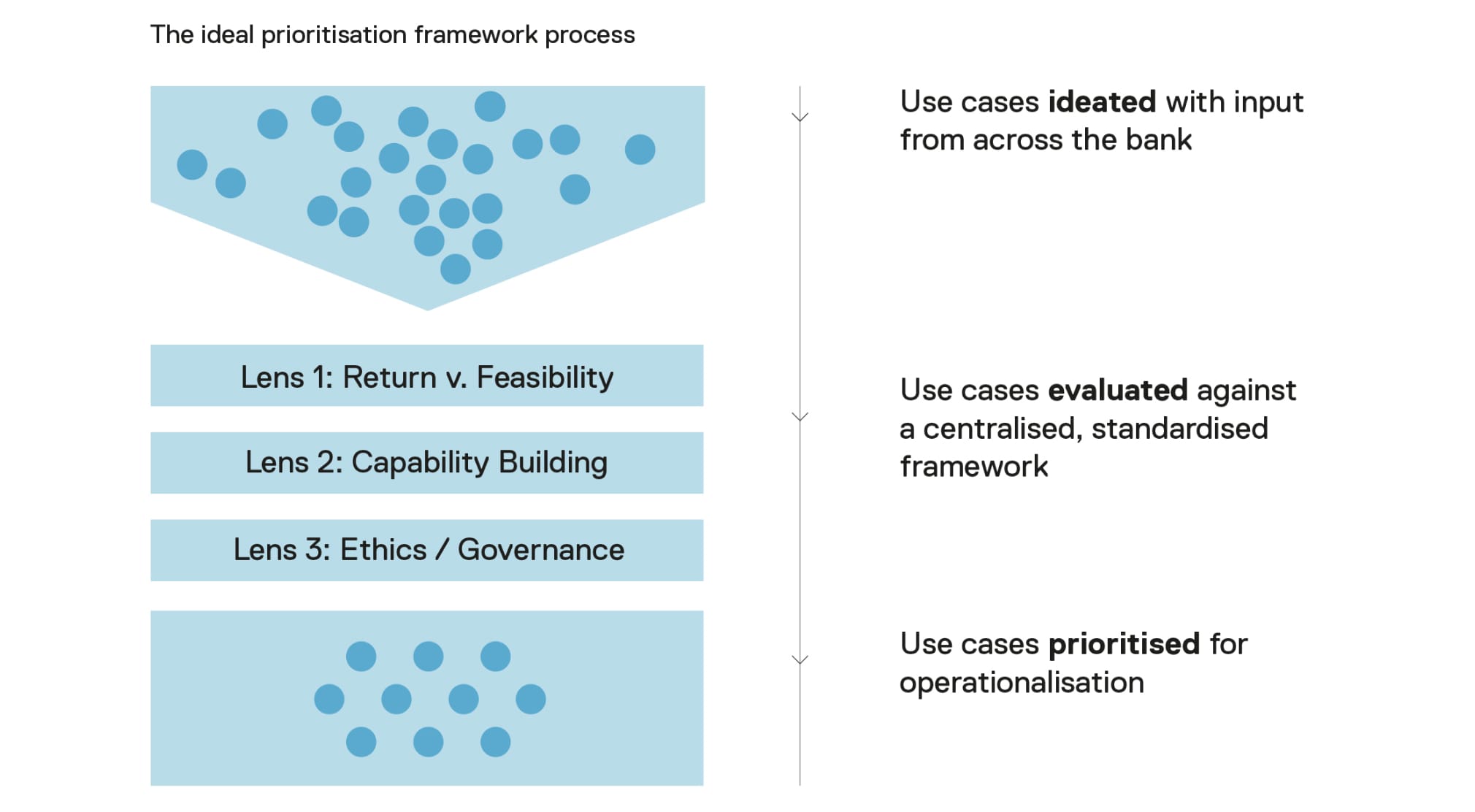
6
Operationalise | Only as use cases are operationalised can banks unlock the value of AI, and incrementally build on success throughout the organisation.
Delivering value from AI at scale requires foundations to be well laid. Banks are focused on building foundational AI tools; delivering long-term data strategy; establishing external partnerships; and building fit-for-purpose model validation frameworks.
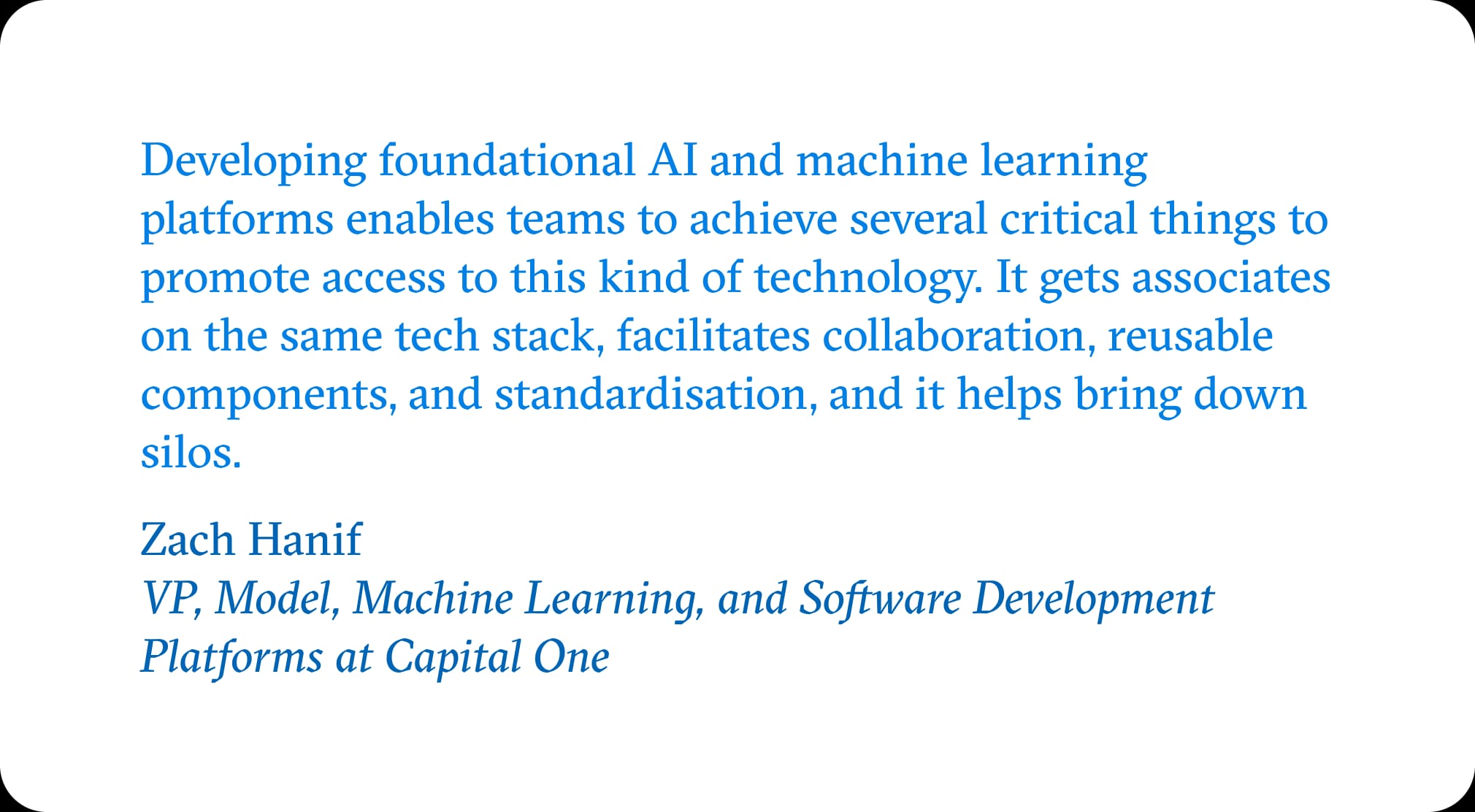
Download the report
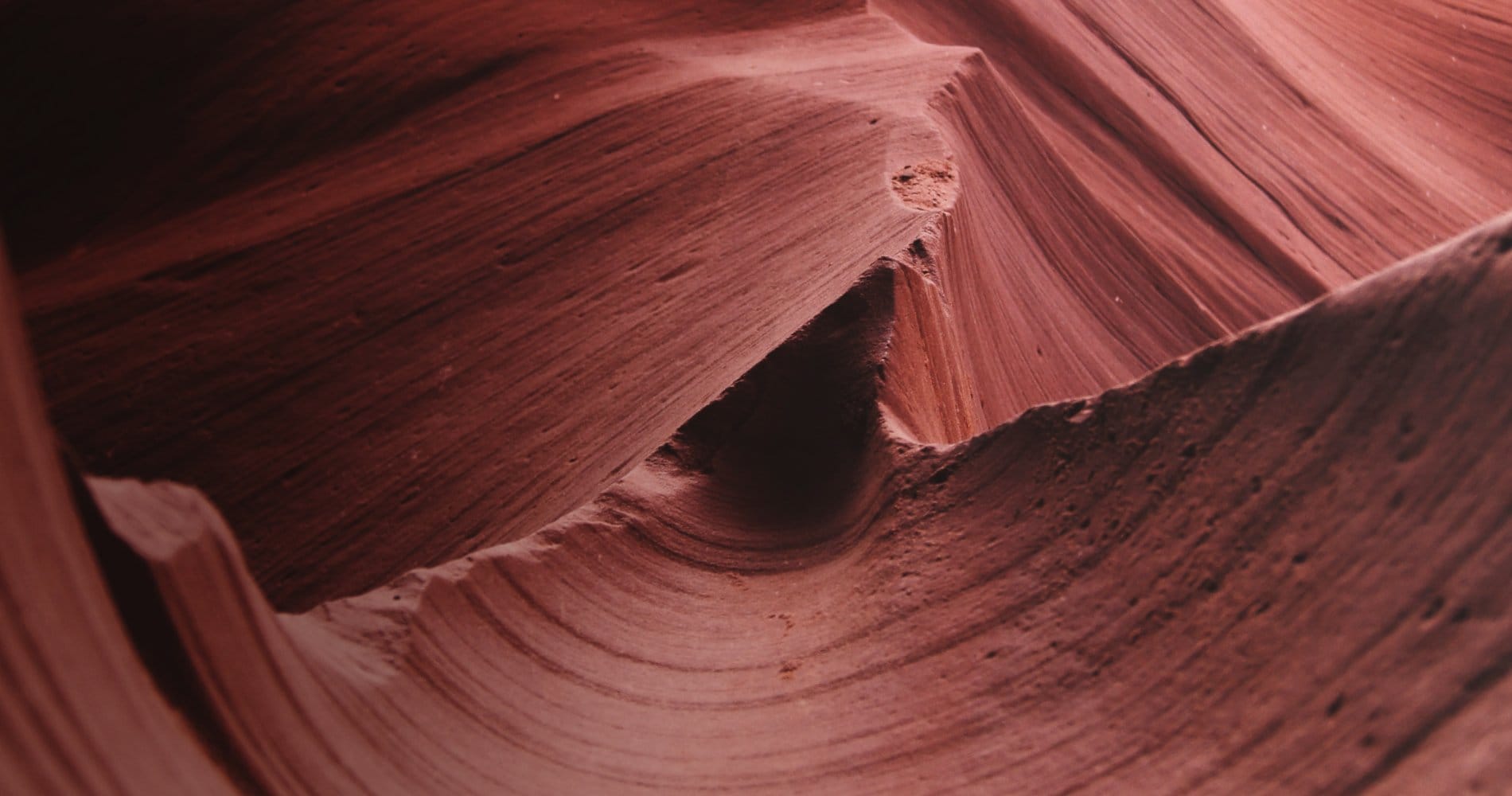
Outcomes Report
The Evident AI Outcomes Report
As the race for AI adoption in banking intensifies, the Evident AI Outcomes Report examines how banks are setting themselves up to drive, and accelerate, value from AI. We explore the emerging best practice among banks for understanding the AI opportunity; measuring success; ideating use cases; prioritising their development; and operationalising those use cases to deliver results. It marks the first step towards benchmarking AI outcomes across the sector. Our ambition is to provide a common framework which will enable banks to evaluate and compare their AI outcomes vs peers.
Get in touch
Related Products